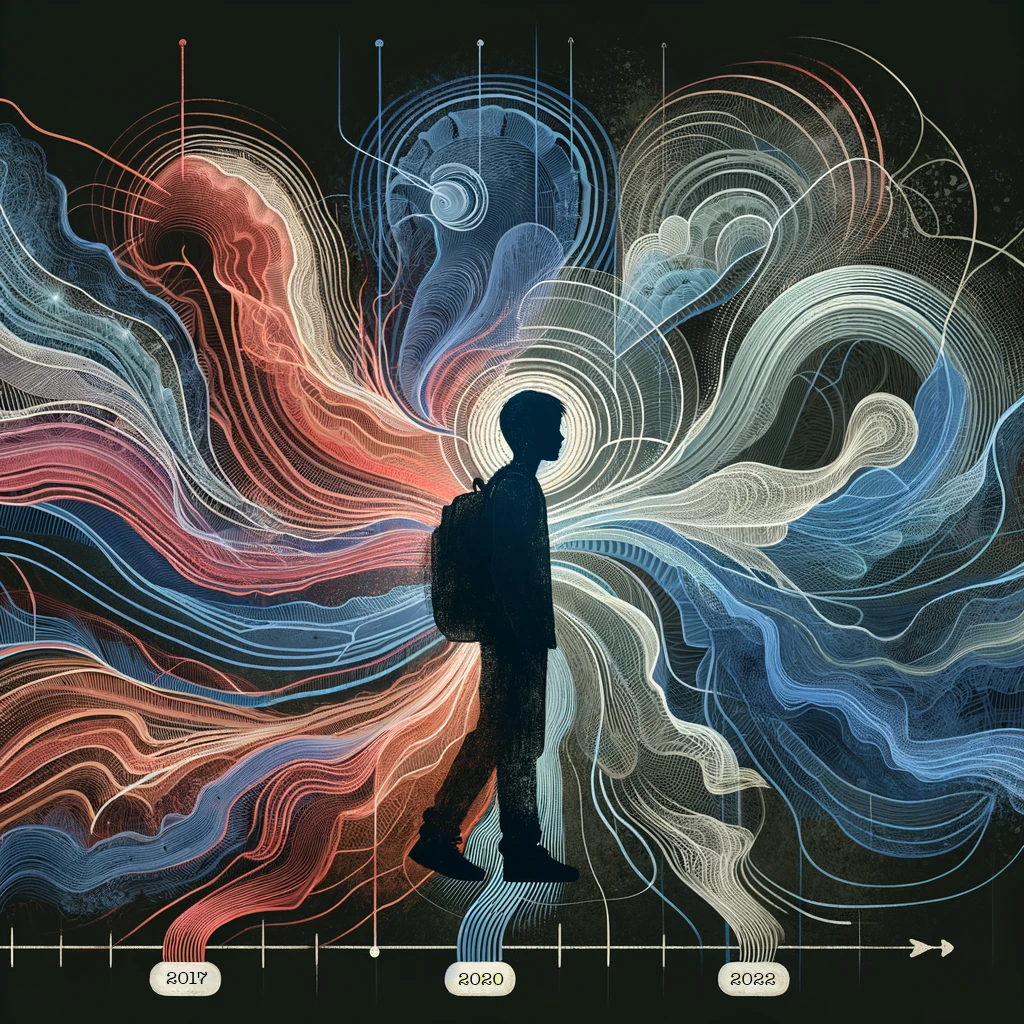
Ebb and Flow of Mental Health Throughout College Years: We track two cohorts of first-year students at Dartmouth College for four years, both on and off campus, creating the longest longitudinal mobile sensing study to date.
The StudentLife study at Dartmouth College is driven by the idea that smartphones, wearables, machine learning, and AI technology will bridge the gap between the skyrocketing demand for mental health support on our campuses and the limited access to healthcare professionals. Moreover, we believe AI will revolutionize diagnosing, managing, and treating mental illness at scale, on our campus and worldwide.
In 2013, we initiated the original StudentLife study at Dartmouth College to capture the hidden stress and strain across a single academic term of 48 undergraduate students, using smartphones as human behavioral sensors (see results). In 2016, we conducted a study with 83 students over two terms using smartphones and wearables to investigate the dynamics of depression and anxiety (see results). In 2018, we tracked 200 high school students throughout their four years at Dartmouth College, which included the challenging period of the COVID-19 pandemic (see results).
While this site primarily focuses on reporting the use of the StudentLife app within undergraduate student populations at Dartmouth College, it is worth noting that the StudentLife app has also been employed in numerous studies worldwide. These studies extend beyond student populations and include clinical research on conditions such as depression, bipolar disorder, anxiety, and severe mental illnesses, including schizophrenia (see papers).
StudentLife is the first study that uses passive and automatic sensing data from the phones of a class of 48 Dartmouth students over a 10 week term to assess their mental health (e.g., depression, loneliness, stress), academic performance (grades across all their classes, term GPA and cumulative GPA) and behavioral trends (e.g., how stress, sleep, visits to the gym, etc. change in response to college workload -- i.e., assignments, midterms, finals -- as the term progresses).
Much of the stress and strain of student life remains hidden. In reality faculty, student deans, clinicians know little about their students outside of the classroom. Students might know about their own circumstances and patterns but know little about classmates. To shine a light on student life we develop the first of a kind StudentLife smartphone app and sensing system to automatically infer human behavior. Why do some students do better than others? Under similar conditions, why do some individuals excel while others fail? Why do students burnout, drop classes, even drop out of college? What is the impact of stress, mood, workload, sociability, sleep and mental health on academic performance (i.e., GPA)? The study used an android app we developed for smartphones carried by 48 students over a 10 week term to find answers to some of these pressing questions.
We use computational methods and machine learning algorithms on the phone to assess sensor data and make higher level inferences (i.e., sleep, sociability, activity, etc.) The StudentLife app that ran on students' phones automatically measured the following human behaviors 24/7 without any user interaction:
We used a number of well-known pre and post mental health surveys and spring and cumulative GPA as ground truth for evaluation of mental-health and academic performance, respectively.
Below you will find papers that report on some of the findings from the StudentLife dataset. Because we are interested in spurring work in mining human behavior we have released an anonymized version of the StudentLife dataset. Click below to learn more about the dataset.
Feel free to contact us if you have any questions relating to the project, findings or dataset.
The StudentLife study reveals several new insights into college students' lives. Our research has uncovered the unique rhythms of academic terms, shedding light on how students' behaviors and wellbeing fluctuate throughout a semester. From the effects of COVID-19 on mental health and everyday behavior to the academic and social patterns distinguishing first-generation students, our findings offer invaluable perspectives on college life. This study enhances our understanding of the student experience and opens doors to new approaches for supporting student success.
Below, we highlight some key findings, but we encourage you to explore our Publications for a comprehensive overview of our extensive research results.
Building on the original StudentLife study from 2013, we tracked over 200 Dartmouth undergraduates over their entire four-year college journey through momentary assessments, annual surveys, behavioral sensing data, and brain scans. This extensive dataset covers the period from 2017 to 2022, which includes timeframes before, during, and immediately following the COVID-19 pandemic, establishing it as one of the most prolonged mobile sensing studies focused on investigating changes in mental health to date. We are now releasing the de-identified College Experience Study dataset to facilitate further research.
Ebb and Flow of Mental Health Throughout College Years: We track two cohorts of first-year students at Dartmouth College for four years, both on and off campus, creating the longest longitudinal mobile sensing study to date.
First Gen Lens: We discuss risk factors, behavioral patterns and mental health of first-generation and non-first-generation students. We propose a deep learning model that accurately predicts the mental health of first-generation students.
Impact of COVID-19 Pandemic: We study the mental health and behavior of college students during the early phases of the COVID-19 Pandemic using longitudinal smartphone sensing and ecological momentary assessments.
Dartmouth Term Rhythm: There is a signature to the Dartmouth term. If we gave StudentLife to all students at Dartmouth we'd find a common rhythm to the intense Dartmouth term.
Predicting GPA with Behavior Sensing: Our model predicts GPA scores that strongly correlate with the ground truth from students’ transcripts (r = 0.81) all within ±0.179 of the reported grades.
What's next? In the future, we hope StudentLife will help students boost their academic performance and wellbeing while living a balanced life on campus.
If you have any questions regarding the study or dataset contact andrew.t.p.campbell [at] gmail [dot] com